NEWS
2024
Paper - "XPL: A Cross-Model framework for Semi-Supervised Prompt Learning in Vision-Language Models" accepted in TMLR 2024.
2023
Was awarded the prestigious Google Fellowship.
Paper - "AnyDA: Anytime Domain Adaptation" accepted in ICLR 2023.
2022
Organized CVPR 2022 Workshop on Dynamic Neural Networks Meets Computer Vision (DNetCV).
2021
Paper - "Semi-Supervised Action Recognition with Temporal Contrastive Learning" accepted in CVPR 2021.
Organized CVPR 2021 Workshop on Dynamic Neural Networks Meets Computer Vision (DNetCV).
RECENT PUBLICATIONS
2024
XPL: A Cross-Model framework for Semi-Supervised Prompt Learning in Vision-Language Models

Prompt learning, which focuses on learning soft prompts, has emerged as a promising approach for efficiently adapting pretrained vision-language models (VLMs) to multiple downstream tasks. While prior works have shown promising performances on common benchmarks, they typically rely on labeled data samples only. This greatly discredits the information gain from the vast collection of otherwise unlabeled samples available in the wild. To mitigate this, we propose a simple yet efficient cross-model framework to leverage on the unlabeled samples achieving significant gain in model performance. Specifically, we employ a semi-supervised prompt learning approach which makes the learned prompts invariant to the different views of a given unlabeled sample. The multiple views are obtained using different augmentations on the images as well as by varying the lengths of visual and text prompts attached to these samples. Experimenting with this simple yet surprisingly effective approach over a large number of benchmark datasets, we observe a considerable improvement in the quality of soft prompts thereby making an immense gain in image classification performance. Interestingly, our approach also benefits from out-of-domain unlabeled images highlighting the robustness and generalization capabilities.
2023
AnyDA: Anytime Domain Adaptation
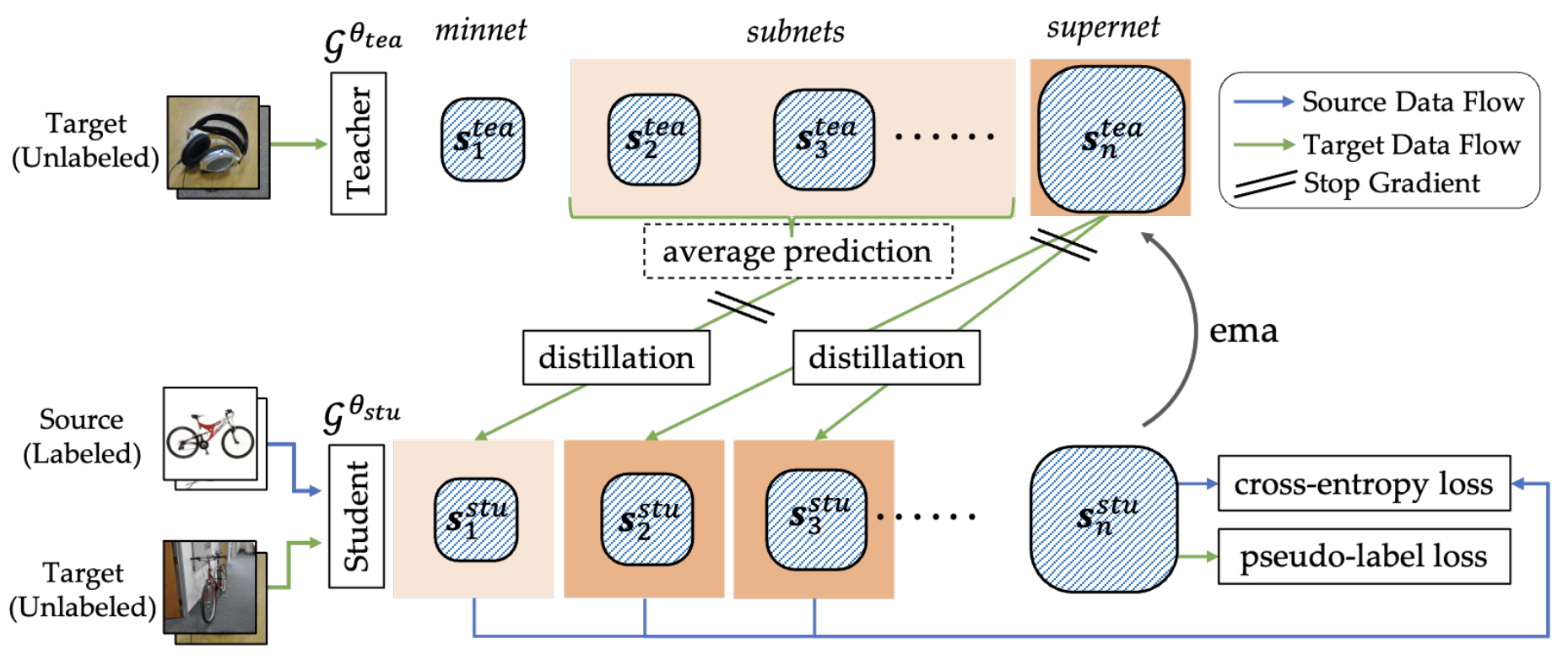
Unsupervised domain adaptation is an open and challenging problem in computer vision. While existing research shows encouraging results in addressing cross-domain distribution shift on common benchmarks, they are often limited to testing under a specific target setting. This can limit their impact for many real-world applications that present different resource constraints. In this paper, we introduce a simple yet effective framework for anytime domain adaptation that is executable with dynamic resource constraints to achieve accuracy-efficiency trade-offs under domain-shifts. We achieve this by training a single shared network using both labeled source and unlabeled data, with switchable depth, width and input resolutions on the fly to enable testing under a wide range of computation budgets. Starting with a teacher network trained from a label-rich source domain, we utilize bootstrapped recursive knowledge distillation as a nexus between source and target domains to jointly train the student network with switchable subnetworks. Extensive experiments on several diverse benchmark datasets well demonstrate the superiority of our proposed approach over state-of-the-art methods.
2021
Semi-Supervised Action Recognition with Temporal Contrastive Learning
[Project] [Code] [Poster] [video presentation]
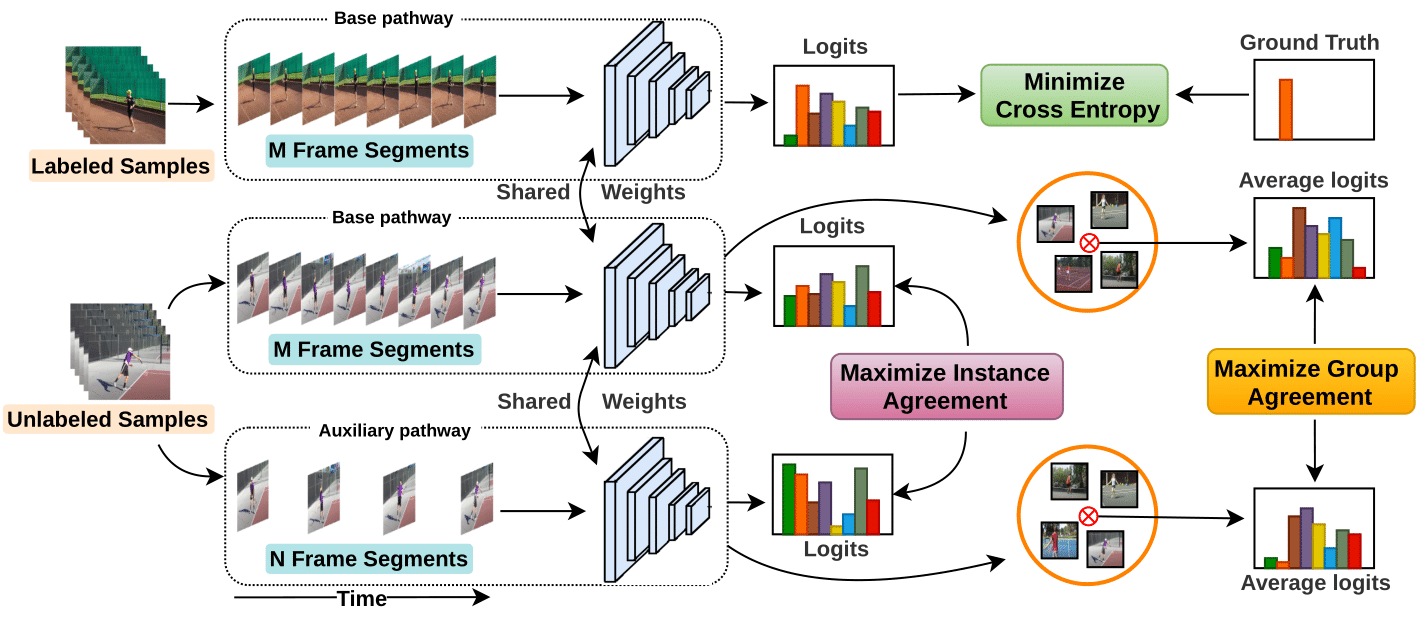
Learning to recognize actions from only a handful of labeled videos is a challenging problem due to the scarcity of tediously collected activity labels. We approach this problem by learning a two-pathway temporal contrastive model using unlabeled videos at two different speeds leveraging the fact that changing video speed does not change an action. Specifically, we propose to maximize the similarity between encoded representations of the same video at two different speeds as well as minimize the similarity between different videos played at different speeds. This way we use the rich supervisory information in terms of ‘time’ that is present in otherwise unsupervised pool of videos. With this simple yet effective strategy of manipulating video playback rates, we considerably outperform video extensions of sophisticated state-of-the-art semi-supervised image recognition methods across multiple diverse benchmark datasets and network architectures. Interestingly, our proposed approach benefits from out-of-domain unlabeled videos showing generalization and robustness. We also perform rigorous ablations and analysis to validate our approach.